Masked autoencoders (MAE) are scalable self-supervised learners for computer vision.
Masked Autoencoders Are Scalable Vision Learners
Approach: MAE mask random patches of the input image and reconstruct the missing pixels. It is based on two core designs.
- Asymmetric encoder-decoder architecture:
- Encoder is a ViT but operates only on the visible unmasked patches. Encoder embeds patches by a linear projection with added positional embeddings, and then processes the resulting set via a series of Transformer blocks.
- Decoder that reconstructs the original image. The input to the MAE decoder is the full set of tokens consisting of (i) encoded visible patches, and (ii) mask tokens. Add positional embeddings to all tokens in this full set; without this, mask tokens would have no information about their location in the image. The decoder has another series of Transformer blocks.
- The MAE decoder is only used during pre-training to perform the image reconstruction task (only the encoder is used to produce image representations for recognition). Therefore, the decoder architecture can be flexibly designed in a manner that is independent of the encoder design.
- Reconstruction target.
- Masking a high proportion of the input image, e.g., 75%, yields a nontrivial and meaningful self-supervisory task.
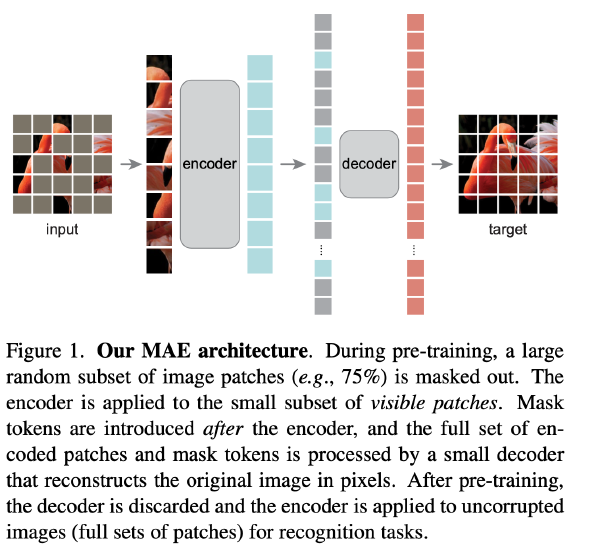
Introduction:
what makes masked autoencoding different between vision and language?
- Convolutions not straightforward to integrate ‘indicators’ such as mask tokens or positional embeddings → ViT
- Information density is different between language and vision. Masking a very high portion of random patches largely reduces redundancy and creates a challenging self- supervisory task that requires holistic understanding beyond low-level image statistics.
- In vision, the decoder reconstructs pixels, hence its output is of a lower semantic level than common recognition tasks; different from BERT, where the decoder predicts missing words that contain rich semantic information.
Related Work
- Masked language modeling and its autoregressive counterparts, e.g., BERT [14] and GPT
- Autoencoding has an encoder that maps an input to a latent representation and a decoder that reconstructs the input. MAE is a form of denoising autoencoding
- Masked image encoding methods learn representations from images corrupted by masking.
- Self-supervised learning, contrastive and related methods strongly depend on data augmentation.