The simple pre-training task of predicting which caption goes with which image is an efficient and scalable way to learn SOTA image representations from scratch. After pre-training, natural language is used to reference learned visual concepts (or describe new ones) enabling zero-shot transfer of the model to downstream tasks.
Learning Transferable Visual Models From Natural Language Supervision
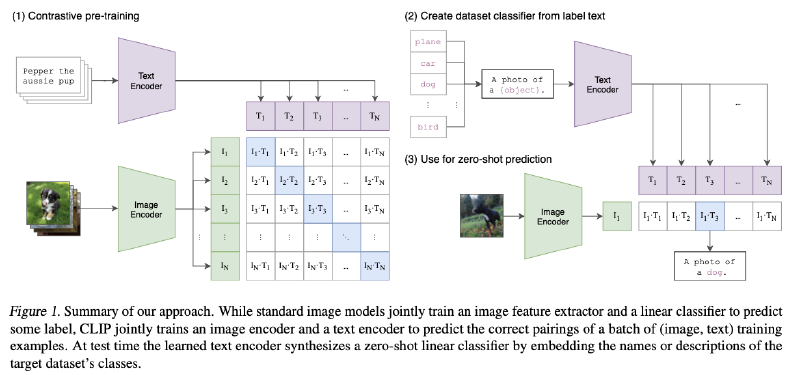
Introduction & Motivation:
- Zero-shot transfer: “text-to-text” as a standardized input-output interface has enabled task-agnostic architectures to zero-shot transfer to downstream datasets removing the need for specialized output heads or dataset specific customization. E.g. GPT3, BERT.
- Learn image representations from text: VirTex, ICMLM, and ConVIRT showed the potential of transformer-based language modeling, masked language modeling, and contrastive objectives to learn image representations from text.
- Scale: weak supervision have improved performance. But crucial difference between weakly supervised models and learning image representations directly from natural language is scale.
Clip(Contrastive Language-Image Pre-training): create a new dataset of 400 million (image, text) pairs and a simplified version of ConVIRT trained from scratch is an efficient method of learning from natural language supervision.
- CLIP outperforms the best publicly available ImageNet model more computationally efficient.
- Zero-shot CLIP models more robust than equivalent accuracy supervised ImageNet models → zero-shot evaluation of task-agnostic models is much more representative of a model’s capability.
Strengths of learning from natural language:
- Easier to scale natural language supervision compared to standard crowd-sourced labeling for image classification since it does not require annotations
- Doesn’t “just” learn a representation but also connects that representation to language which enables flexible zero-shot transfer.
Selecting an Efficient Pre-Training Method - Contrastive learning
- Given a batch of N (image, text) pairs, CLIP is trained to predict which of the $N x N$ possible (image, text) pairings across a batch actually occurred.
- CLIP learns a multi-modal embedding space by jointly training an image encoder and text encoder to
- Maximize the cosine similarity of the image and text embeddings of the $N$ real pairs in the batch;
- Minimize the cosine similarity of the embeddings of the $N^2 - N$ incorrect pairings.
- Optimize a symmetric cross entropy loss over these similarity scores: *multi-class N-pair loss (*InfoNCE loss).
Choosing and Scaling a Model
- Image Encoder: ResNet-50 & Vision Transformer
- Text Encoder: Transformer
Training
- Trained 5 ResNets(ResNet-50, a ResNet-101, and 3 more follow EfficientNet-style model scaling) and 3 Vision Transformers (ViT-B/32, a ViT-B/16, and a ViT-L/14); Adam optimizer
- To accelerate training and save memory, use:
Mixed-precision (Micikevicius et al., 2017)
Gradient checkpointing (Griewank & Walther, 2000; Chen et al., 2016)
Half-precision Adam statistics (Dhariwal et al., 2020)
Half-precision stochastically rounded text encoder weights
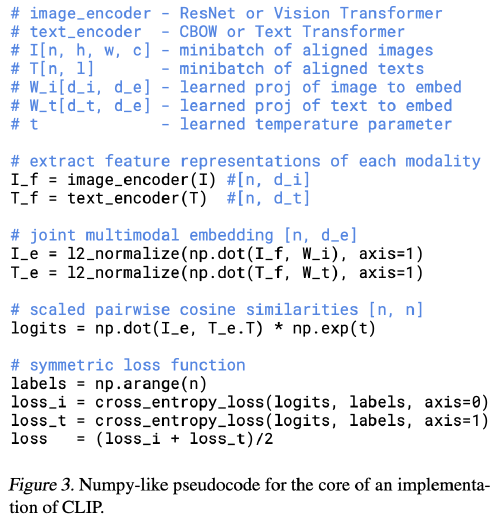
Zero-Shot Transfer
- Zero-shot learning: generalizing to unseen object categories in image classification.
- We motivate studying zero-shot transfer as a way of measuring the task-learning capabilities of machine learning systems.
- Using CLIP for zero-shot transfer:
- Compute the feature embedding of the image and the feature embedding of the set of possible texts by their respective encoders.
- The cosine similarity of these embeddings is then calculated, scaled by a temperature parameter, and normalized via a softmax.
- For zero-shot evaluation, we cache the zero-shot classifier once it has been computed by the text encoder and reuse it for all subsequent predictions.
- Prompt engineering and ensembling
Issues of using one-word labels:
- Polysemy (多义性);
- Distribution gap: rare in our pre-training dataset for the text paired with the image to be just a single word. Usually the text is a full sentence describing the image in some way.
Solution: prompt template “A photo of a flabelg.”is a good default that helps specify the text is about the content of the image.
- Improve zero-shot performance by customizing the prompt text to each task.
Better solution: Ensembling over multiple zeroshot classifiers using different context prompt.多用几个模版然后把结果结合
Reference:
- Code: https://github.com/openai/CLIP
- Paper: https://arxiv.org/abs/2103.00020
- Notebook: https://colab.research.google.com/github/openai/clip/blob/master/notebooks/Interacting_with_CLIP.ipynb
- Blog: https://openai.com/research/clip
- Paper walkthrough: https://www.bilibili.com/video/BV1SL4y1s7LQ/?spm_id_from=333.788&vd_source=d413f9c111fd65e9e19a68a23199ac36